The Ultimate Guide to Data Annotation Tools and Platforms
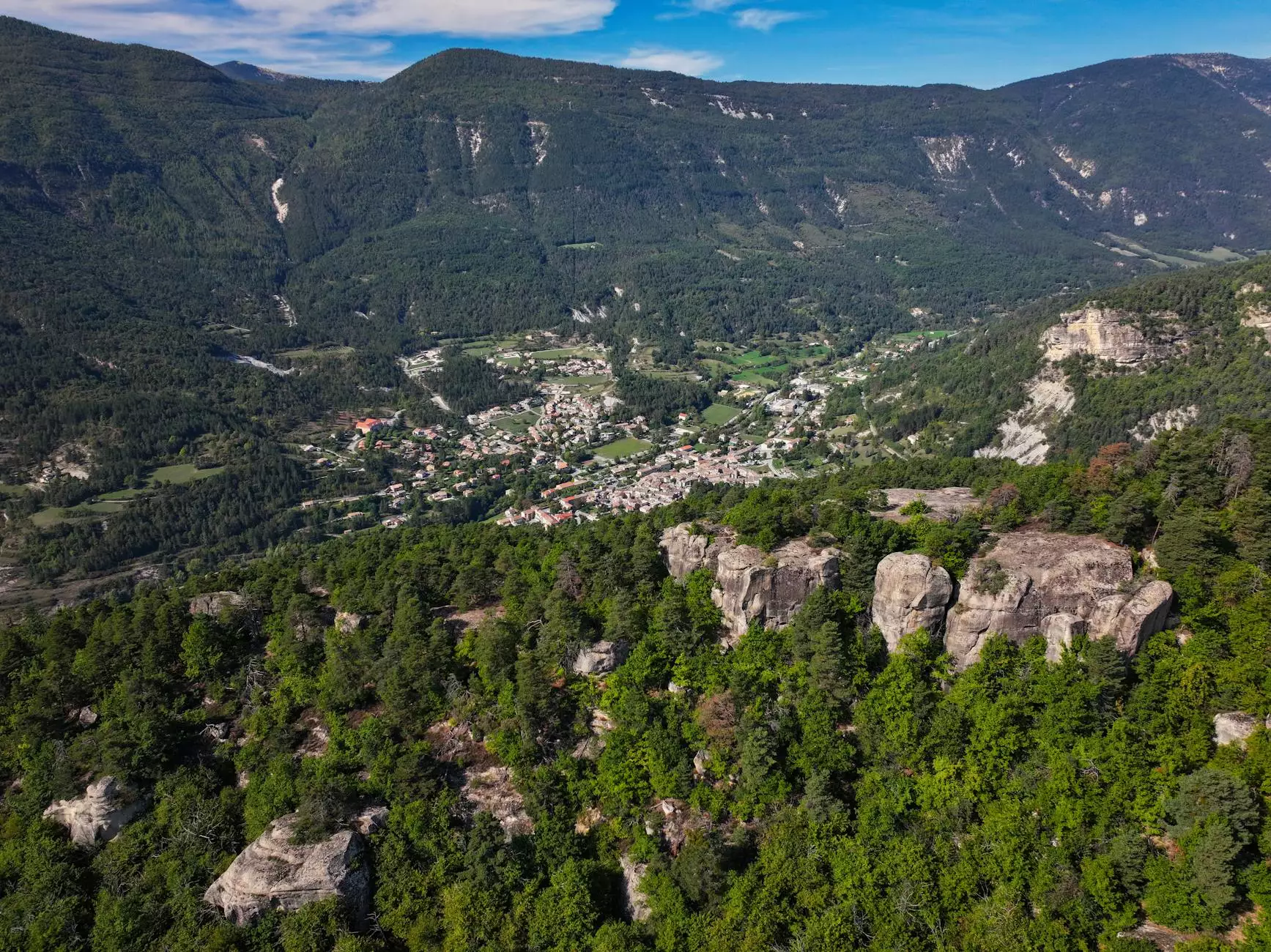
In a world increasingly driven by data, the importance of data annotation cannot be overstated. Businesses across various sectors, from healthcare to automotive, are leveraging artificial intelligence (AI) and machine learning (ML) to compete in the modern landscape. A crucial element in training effective machine learning models is the data annotation process. This comprehensive article explores the best practices, methodologies, and tools available in the domain of data annotation, focusing significantly on platforms like KeyLabs.ai.
What is Data Annotation?
Data annotation is the process of adding metadata to raw data (like images, text, or audio) to make it understandable for machine learning algorithms. This process is vital for the supervised learning model, where the algorithm learns by training on labeled datasets. The significance of accurate and effective data annotation cannot be ignorant in today’s data-driven environment. It serves as the backbone for teaching machine learning models to make predictions and decisions based on patterns and insights derived from data.
Types of Data Annotation
1. Image Annotation
Image annotation involves adding labels to images. Common techniques include:
- Bounding Boxes: This technique is used to identify the object of interest in an image by drawing a rectangle around it.
- Segmentation: Segmentation annotations involve delineating the different segments of an image.
- Landmarking: In this method, key points in the image are labeled to aid in understanding object orientation.
2. Video Annotation
Video annotation requires an extension of image annotation techniques, as it involves labeling frames in a sequence to capture motion and behavior over time.
3. Text Annotation
This involves tagging text with relevant information. Common forms of text annotation include:
- Entity Recognition: Identifying and classifying key pieces of information (like names, dates, and locations).
- Sentiment Analysis: Tagging pieces of text based on the emotional tone they convey.
4. Audio Annotation
Audio annotations typically involve labeling spoken phrases in a recording, identifying speakers, and noting background sounds to improve natural language processing (NLP) systems.
The Importance of Data Annotation
Data annotation plays an essential role in:
- Improving Model Accuracy: Labeled data provides clarity and precision, which translates to more accurate predictions by machine learning models.
- Enabling Effective Learning: Each piece of annotated data serves as a learning point for the algorithm, helping it understand nuances and make better decisions over time.
- Facilitating Automation: Annotated datasets allow for the automation of tasks that require a high level of precision, paving the way for innovations across various industries.
Choosing the Right Data Annotation Tool
When selecting a data annotation tool or platform, consider the following criteria to enhance effectiveness and efficiency:
- Ease of Use: The user interface should be intuitive and easily navigable.
- Scalability: Capable of handling increasing volumes of data as your project grows.
- Collaboration Features: Ability to facilitate teamwork, especially if multiple annotators are involved.
- Quality Assurance: Features that ensure high-quality annotations (like review functions).
- Integration Capabilities: Ability to integrate with other tools and platforms for smooth workflows.
Overview of KeyLabs.ai
Among the leading pioneers in the realm of data annotation is KeyLabs.ai. This platform provides a comprehensive array of solutions aimed at optimizing the data annotation process. KeyLabs.ai is characterized by:
- Advanced Technology: Utilizing AI-driven tools to enhance and accelerate the annotation process.
- User-Friendly Interface: Its platform is designed for ease of use, facilitating seamless navigation for users of all skill levels.
- Custom Solutions: Offers tailor-made solutions to fit various industry needs and data types.
- Expert Support: Provides expert guidance and support to ensure clients achieve their data annotation goals efficiently.
Benefits of Using KeyLabs.ai for Data Annotation
Choosing KeyLabs.ai for your data annotation needs can provide your business with a competitive edge. Here are some key benefits:
1. Enhanced Data Quality
With expert human annotators and machine learning assistance, the quality of data annotations produced is remarkably high. This dramatically increases the accuracy of models trained on such datasets.
2. Speed and Efficiency
KeyLabs.ai employs sophisticated tools that expedite the annotation process, ensuring faster turnaround times without compromising quality. This is particularly crucial in dynamic markets where quick data response can dictate success.
3. Cost-Effective Solutions
Leveraging automated processes combined with human oversight helps in producing cost-effective solutions. Businesses can allocate resources more efficiently while still achieving stellar outcomes.
4. Flexibility and Customization
Regardless of the industry or data types, KeyLabs.ai offers customizable solutions that cater to specific project needs, allowing businesses to scale operations efficiently.
5. Collaboration and Communication
The platform ensures that teams can work together effectively through integrated communication tools, thus enhancing the consensus on annotation protocols and guidelines.
Best Practices for Data Annotation
To truly harness the power of data annotation tools and platforms like KeyLabs.ai, businesses should adhere to the following best practices:
- Define Clear Objectives: Understanding the end goal of the data annotation process is crucial for ensuring relevancy and accuracy in the annotations created.
- Establish Annotation Guidelines: Clear guidelines should be crafted and disseminated to all annotators to maintain consistency across the dataset.
- Utilize a Hybrid Approach: Combining AI with human annotation can result in higher quality outputs, as machines can rapidly annotate while humans can adjust for nuances missed by AI.
- Implement Continuous Feedback Loops: Regularly reviewing annotations and providing feedback can lead to continuous improvement in both the annotation process and the quality of the datasets.
- Invest in Training: Keeping your annotators well-trained in the latest technologies and trends in data annotation is a step towards maintaining quality and efficiency.
Conclusion
Data annotation stands as an indispensable pillar supporting the development and success of machine learning and AI applications. This guide underscores the importance of utilizing robust platforms like KeyLabs.ai, which streamlines the annotation process, ensuring high-quality outputs that can propel businesses forward in their digital transformation journeys.
As we continue to navigate through an ever-evolving data landscape, understanding the nuances of data annotation will be vital for any business aiming to harness the power of AI and machine learning. With the right tools and practices, businesses can ensure they are well-equipped to meet the challenges of tomorrow and leverage the vast potential that data has to offer.
https://keylabs.ai/